Standardize metadata on-the-fly#
This use cases runs on a LaminDB instance with populated CellType
and Pathway
registries. Make sure you run the GO Ontology notebook before executing this use case.
Here, we demonstrate how to standardize the metadata on-the-fly during cell type annotation and pathway enrichment analysis using these two registries.
For more information, see:
!lamin load use-cases-registries
π‘ found cached instance metadata: /home/runner/.lamin/instance--testuser1--use-cases-registries.env
π‘ connected lamindb: testuser1/use-cases-registries
import lamindb as ln
import bionty as bt
from lamin_usecases import datasets as ds
import scanpy as sc
import matplotlib.pyplot as plt
import celltypist
import gseapy as gp
π‘ connected lamindb: testuser1/use-cases-registries
sc.settings.set_figure_params(dpi=50, facecolor="white")
ln.transform.stem_uid = "hsPU1OENv0LS"
ln.transform.version = "0"
ln.track()
π‘ notebook imports: bionty==0.42.4 celltypist==1.6.2 gseapy==1.1.2 lamin_usecases==0.0.1 lamindb==0.69.2 matplotlib==3.8.3 scanpy==1.10.0
π‘ saved: Transform(uid='hsPU1OENv0LS6K79', name='Standardize metadata on-the-fly', key='analysis-registries', version='0', type=notebook, updated_at=2024-03-28 10:22:41 UTC, created_by_id=1)
π‘ saved: Run(uid='SebWd1hkmKyITL1cxdEa', transform_id=1, created_by_id=1)
An interferon-beta treated dataset#
A small peripheral blood mononuclear cell dataset that is split into control and stimulated groups. The stimulated group was treated with interferon beta.
Letβs load the dataset and perform some preprocessing:
adata = ds.anndata_seurat_ifnb(preprocess=False, populate_registries=True)
adata
AnnData object with n_obs Γ n_vars = 13999 Γ 9936
obs: 'stim'
var: 'symbol'
sc.pp.normalize_total(adata, target_sum=1e4)
sc.pp.log1p(adata)
sc.pp.highly_variable_genes(adata, n_top_genes=2000)
sc.pp.pca(adata, n_comps=20)
sc.pp.neighbors(adata, n_pcs=10)
sc.tl.umap(adata)
Analysis: cell type annotation using CellTypist#
model = celltypist.models.Model.load(model="Immune_All_Low.pkl")
Show code cell output
2024-03-28 10:23:53,699:INFO - π No available models. Downloading...
2024-03-28 10:23:53,700:INFO - π Retrieving model list from server https://celltypist.cog.sanger.ac.uk/models/models.json
2024-03-28 10:24:02,833:INFO - π Total models in list: 44
2024-03-28 10:24:02,834:INFO - π Storing models in /home/runner/.celltypist/data/models
2024-03-28 10:24:02,835:INFO - πΎ Downloading model [1/44]: Immune_All_Low.pkl
2024-03-28 10:24:03,975:INFO - πΎ Downloading model [2/44]: Immune_All_High.pkl
2024-03-28 10:24:05,100:INFO - πΎ Downloading model [3/44]: Adult_CynomolgusMacaque_Hippocampus.pkl
2024-03-28 10:24:05,970:INFO - πΎ Downloading model [4/44]: Adult_Human_PancreaticIslet.pkl
2024-03-28 10:24:11,549:INFO - πΎ Downloading model [5/44]: Adult_Human_Skin.pkl
2024-03-28 10:24:17,223:INFO - πΎ Downloading model [6/44]: Adult_Mouse_Gut.pkl
2024-03-28 10:24:23,410:INFO - πΎ Downloading model [7/44]: Adult_Mouse_OlfactoryBulb.pkl
2024-03-28 10:24:24,334:INFO - πΎ Downloading model [8/44]: Adult_Pig_Hippocampus.pkl
2024-03-28 10:24:25,076:INFO - πΎ Downloading model [9/44]: Adult_RhesusMacaque_Hippocampus.pkl
2024-03-28 10:24:25,818:INFO - πΎ Downloading model [10/44]: Autopsy_COVID19_Lung.pkl
2024-03-28 10:24:26,663:INFO - πΎ Downloading model [11/44]: COVID19_HumanChallenge_Blood.pkl
2024-03-28 10:24:27,597:INFO - πΎ Downloading model [12/44]: COVID19_Immune_Landscape.pkl
2024-03-28 10:24:28,436:INFO - πΎ Downloading model [13/44]: Cells_Fetal_Lung.pkl
2024-03-28 10:24:29,605:INFO - πΎ Downloading model [14/44]: Cells_Intestinal_Tract.pkl
2024-03-28 10:24:30,740:INFO - πΎ Downloading model [15/44]: Cells_Lung_Airway.pkl
2024-03-28 10:24:31,687:INFO - πΎ Downloading model [16/44]: Developing_Human_Brain.pkl
2024-03-28 10:24:37,538:INFO - πΎ Downloading model [17/44]: Developing_Human_Gonads.pkl
2024-03-28 10:24:38,472:INFO - πΎ Downloading model [18/44]: Developing_Human_Hippocampus.pkl
2024-03-28 10:24:39,239:INFO - πΎ Downloading model [19/44]: Developing_Human_Organs.pkl
2024-03-28 10:24:40,070:INFO - πΎ Downloading model [20/44]: Developing_Human_Thymus.pkl
2024-03-28 10:24:44,879:INFO - πΎ Downloading model [21/44]: Developing_Mouse_Brain.pkl
2024-03-28 10:24:46,074:INFO - πΎ Downloading model [22/44]: Developing_Mouse_Hippocampus.pkl
2024-03-28 10:24:46,820:INFO - πΎ Downloading model [23/44]: Fetal_Human_AdrenalGlands.pkl
2024-03-28 10:24:47,466:INFO - πΎ Downloading model [24/44]: Fetal_Human_Pancreas.pkl
2024-03-28 10:24:48,318:INFO - πΎ Downloading model [25/44]: Fetal_Human_Pituitary.pkl
2024-03-28 10:24:49,148:INFO - πΎ Downloading model [26/44]: Fetal_Human_Retina.pkl
2024-03-28 10:24:50,092:INFO - πΎ Downloading model [27/44]: Fetal_Human_Skin.pkl
2024-03-28 10:24:50,833:INFO - πΎ Downloading model [28/44]: Healthy_Adult_Heart.pkl
2024-03-28 10:24:51,785:INFO - πΎ Downloading model [29/44]: Healthy_COVID19_PBMC.pkl
2024-03-28 10:24:52,625:INFO - πΎ Downloading model [30/44]: Healthy_Human_Liver.pkl
2024-03-28 10:24:53,389:INFO - πΎ Downloading model [31/44]: Healthy_Mouse_Liver.pkl
2024-03-28 10:24:54,131:INFO - πΎ Downloading model [32/44]: Human_AdultAged_Hippocampus.pkl
2024-03-28 10:24:54,874:INFO - πΎ Downloading model [33/44]: Human_Developmental_Retina.pkl
2024-03-28 10:24:55,610:INFO - πΎ Downloading model [34/44]: Human_Embryonic_YolkSac.pkl
2024-03-28 10:24:56,537:INFO - πΎ Downloading model [35/44]: Human_IPF_Lung.pkl
2024-03-28 10:24:57,396:INFO - πΎ Downloading model [36/44]: Human_Longitudinal_Hippocampus.pkl
2024-03-28 10:24:58,138:INFO - πΎ Downloading model [37/44]: Human_Lung_Atlas.pkl
2024-03-28 10:24:59,085:INFO - πΎ Downloading model [38/44]: Human_PF_Lung.pkl
2024-03-28 10:24:59,920:INFO - πΎ Downloading model [39/44]: Lethal_COVID19_Lung.pkl
2024-03-28 10:25:00,770:INFO - πΎ Downloading model [40/44]: Mouse_Dentate_Gyrus.pkl
2024-03-28 10:25:01,714:INFO - πΎ Downloading model [41/44]: Mouse_Isocortex_Hippocampus.pkl
2024-03-28 10:25:02,544:INFO - πΎ Downloading model [42/44]: Mouse_Postnatal_DentateGyrus.pkl
2024-03-28 10:25:03,474:INFO - πΎ Downloading model [43/44]: Nuclei_Lung_Airway.pkl
2024-03-28 10:25:04,504:INFO - πΎ Downloading model [44/44]: Pan_Fetal_Human.pkl
predictions = celltypist.annotate(
adata, model="Immune_All_Low.pkl", majority_voting=True
)
adata.obs["cell_type_celltypist"] = predictions.predicted_labels.majority_voting
2024-03-28 10:25:05,653:INFO - π¬ Input data has 13999 cells and 9936 genes
2024-03-28 10:25:05,654:INFO - π Matching reference genes in the model
2024-03-28 10:25:06,794:INFO - 𧬠3698 features used for prediction
2024-03-28 10:25:06,798:INFO - βοΈ Scaling input data
2024-03-28 10:25:07,256:INFO - ποΈ Predicting labels
2024-03-28 10:25:07,460:INFO - β
Prediction done!
2024-03-28 10:25:07,463:INFO - π Detected a neighborhood graph in the input object, will run over-clustering on the basis of it
2024-03-28 10:25:07,464:INFO - βοΈ Over-clustering input data with resolution set to 10
2024-03-28 10:25:15,393:INFO - π³οΈ Majority voting the predictions
2024-03-28 10:25:15,448:INFO - β
Majority voting done!
bt.CellType.inspect(adata.obs["cell_type_celltypist"]);
β received 14 unique terms, 13985 empty/duplicated terms are ignored
β 14 terms (100.00%) are not validated for name: Intermediate macrophages, B cells, Tcm/Naive helper T cells, Tem/Effector helper T cells, Tem/Trm cytotoxic T cells, Non-classical monocytes, Regulatory T cells, NK cells, Tcm/Naive cytotoxic T cells, CD16+ NK cells, pDC, DC2, Classical monocytes, DC
detected 2 CellType terms in Bionty as synonyms: 'pDC', 'DC2'
β add records from Bionty to your CellType registry via .from_values()
couldn't validate 14 terms: 'Regulatory T cells', 'CD16+ NK cells', 'Intermediate macrophages', 'pDC', 'DC2', 'NK cells', 'Tcm/Naive helper T cells', 'Non-classical monocytes', 'Tem/Effector helper T cells', 'Tcm/Naive cytotoxic T cells', 'Classical monocytes', 'B cells', 'DC', 'Tem/Trm cytotoxic T cells'
β if you are sure, create new records via ln.CellType() and save to your registry
adata.obs["cell_type_celltypist"] = bt.CellType.standardize(
adata.obs["cell_type_celltypist"]
)
β found 2 synonyms in Bionty: ['pDC', 'DC2']
please add corresponding CellType records via `.from_values(['plasmacytoid dendritic cell'])`
# Register cell type of found synonym
bt.CellType.from_public(name='plasmacytoid dendritic cell').save()
β now recursing through parents: this only happens once, but is much slower than bulk saving
sc.pl.umap(
adata,
color=["cell_type_celltypist", "stim"],
frameon=False,
legend_fontsize=10,
wspace=0.4,
)
... storing 'cell_type_celltypist' as categorical
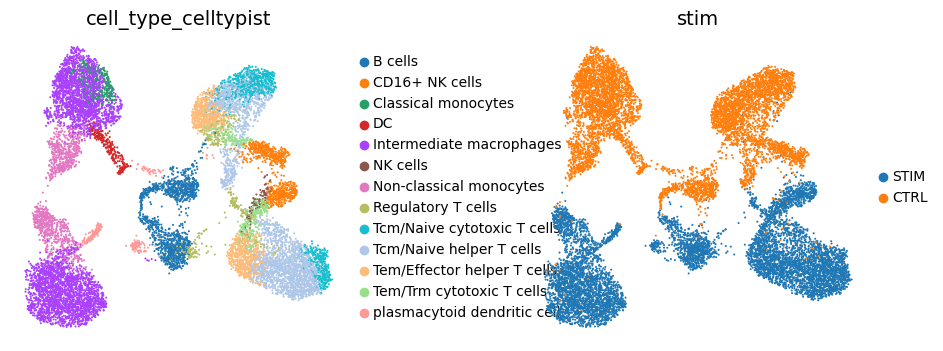
Analysis: Pathway enrichment analysis using Enrichr#
This analysis is based on the GSEApy scRNA-seq Example.
First, we compute differentially expressed genes using a Wilcoxon test between stimulated and control cells.
# compute differentially expressed genes
sc.tl.rank_genes_groups(
adata,
groupby="stim",
use_raw=False,
method="wilcoxon",
groups=["STIM"],
reference="CTRL",
)
rank_genes_groups_df = sc.get.rank_genes_groups_df(adata, "STIM")
rank_genes_groups_df.head()
names | scores | logfoldchanges | pvals | pvals_adj | |
---|---|---|---|---|---|
0 | ISG15 | 99.456726 | 7.132648 | 0.0 | 0.0 |
1 | ISG20 | 96.736618 | 5.074153 | 0.0 | 0.0 |
2 | IFI6 | 94.973267 | 5.828746 | 0.0 | 0.0 |
3 | IFIT3 | 92.482445 | 7.432344 | 0.0 | 0.0 |
4 | IFIT1 | 90.699020 | 8.053449 | 0.0 | 0.0 |
Next, we filter out up/down-regulated differentially expressed gene sets:
degs_up = rank_genes_groups_df[
(rank_genes_groups_df["logfoldchanges"] > 0)
& (rank_genes_groups_df["pvals_adj"] < 0.05)
]
degs_dw = rank_genes_groups_df[
(rank_genes_groups_df["logfoldchanges"] < 0)
& (rank_genes_groups_df["pvals_adj"] < 0.05)
]
degs_up.shape, degs_dw.shape
((541, 5), (937, 5))
Run pathway enrichment analysis on DEGs and plot top 10 pathways:
enr_up = gp.enrichr(degs_up.names, gene_sets="GO_Biological_Process_2023").res2d
gp.dotplot(enr_up, figsize=(2, 3), title="Up", cmap=plt.cm.autumn_r);
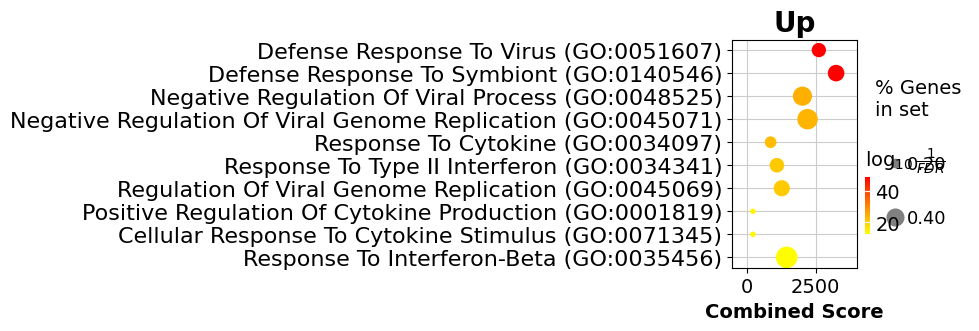
enr_dw = gp.enrichr(degs_dw.names, gene_sets="GO_Biological_Process_2023").res2d
gp.dotplot(enr_dw, figsize=(2, 3), title="Down", cmap=plt.cm.winter_r);
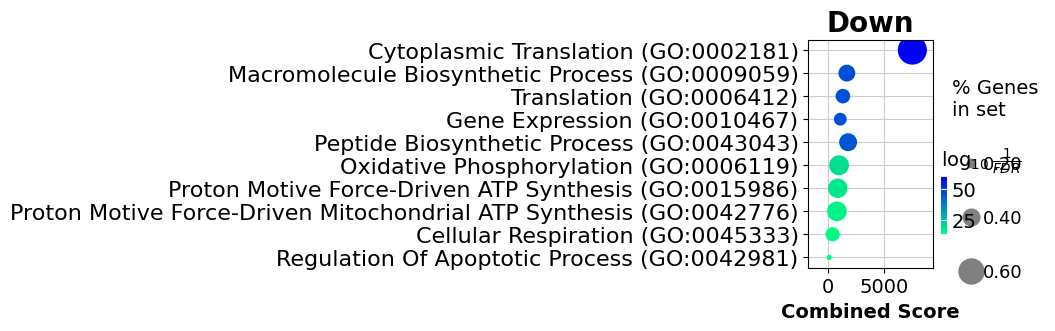
Register analyzed dataset and annotate with metadata#
Register new features and labels (check out more details here):
new_features = ln.Feature.from_df(adata.obs)
ln.save(new_features)
new_labels = [ln.ULabel(name=i) for i in adata.obs["stim"].unique()]
ln.save(new_labels)
features = ln.Feature.lookup()
Register dataset using a Artifact object:
artifact = ln.Artifact.from_anndata(
adata,
description="seurat_ifnb_activated_Bcells",
)
artifact.save()
artifact.features.add_from_anndata(
var_field=bt.Gene.symbol,
organism="human", # optionally, globally set organism via bt.settings.organism = "human"
)
Link cell type labels#
cell_type_records = bt.CellType.from_values(adata.obs["cell_type_celltypist"])
artifact.labels.add(cell_type_records, features.cell_type_celltypist)
β did not create CellType records for 12 non-validated names: 'B cells', 'CD16+ NK cells', 'Classical monocytes', 'DC', 'Intermediate macrophages', 'NK cells', 'Non-classical monocytes', 'Regulatory T cells', 'Tcm/Naive cytotoxic T cells', 'Tcm/Naive helper T cells', 'Tem/Effector helper T cells', 'Tem/Trm cytotoxic T cells'
Link stimulation labels:
stim_records = ln.ULabel.from_values(adata.obs["stim"])
artifact.labels.add(stim_records, features.stim)
Link pathway labels#
Letβs enable tracking of the current notebook as the transform of this artifact:
We further create two feature sets for degs_up
and degs_dw
which we can later associate with the associated pathways:
degs_up_featureset = ln.FeatureSet.from_values(
degs_up.names,
bt.Gene.symbol,
name="Up-regulated DEGs STIM vs CTRL",
type="category",
organism=( # optionally, globally set organism via bt.settings.organism = "human"
"human"
),
)
degs_dw_featureset = ln.FeatureSet.from_values(
degs_dw.names,
bt.Gene.symbol,
name="Down-regulated DEGs STIM vs CTRL",
type="category",
organism=( # optionally, globally set organism via bt.settings.organism = "human"
"human"
),
)
# Link feature sets to artifact
artifact.features._add_feature_set(degs_up_featureset, slot="STIM-up-DEGs")
artifact.features._add_feature_set(degs_dw_featureset, slot="STIM-down-DEGs")
Link the top 10 pathways to the corresponding differentially expressed genes:
def parse_ontology_id_from_enrichr_results(key):
"""Parse out the ontology id.
"ATF6-mediated Unfolded Protein Response (GO:0036500)" -> ("GO:0036500", "ATF6-mediated Unfolded Protein Response")
"""
id = key.split(" ")[-1].replace("(", "").replace(")", "")
name = key.replace(f" ({id})", "")
return (id, name)
# get ontology ids for the top 10 pathways
enr_up_top10 = [
pw_id[0]
for pw_id in enr_up.head(10).Term.apply(parse_ontology_id_from_enrichr_results)
]
enr_dw_top10 = [
pw_id[0]
for pw_id in enr_dw.head(10).Term.apply(parse_ontology_id_from_enrichr_results)
]
# get pathway records
enr_up_top10_pathways = bt.Pathway.from_values(enr_up_top10, bt.Pathway.ontology_id)
enr_dw_top10_pathways = bt.Pathway.from_values(enr_dw_top10, bt.Pathway.ontology_id)
Associate the pathways to the differentially expressed genes:
degs_up_featureset.pathways.set(enr_up_top10_pathways)
degs_dw_featureset.pathways.set(enr_dw_top10_pathways)
degs_up_featureset.pathways.list("name")
['defense response to symbiont',
'response to type II interferon',
'defense response to virus',
'negative regulation of viral genome replication',
'cellular response to cytokine stimulus',
'positive regulation of cytokine production',
'response to interferon-beta',
'negative regulation of viral process',
'response to cytokine',
'regulation of viral genome replication']
Querying metadata#
artifact.describe()
Artifact(uid='iNMvFYSmZNYKZBWcAWJN', suffix='.h5ad', accessor='AnnData', description='seurat_ifnb_activated_Bcells', size=215062782, hash='Py_jXTAbsMikdIQYeoOyGv', hash_type='sha1-fl', visibility=1, key_is_virtual=True, updated_at=2024-03-28 10:25:37 UTC)
Provenance:
ποΈ storage: Storage(uid='0WV4qUGO', root='/home/runner/work/lamin-usecases/lamin-usecases/docs/use-cases-registries', type='local', updated_at=2024-03-28 10:21:44 UTC, created_by_id=1)
π« transform: Transform(uid='hsPU1OENv0LS6K79', name='Standardize metadata on-the-fly', key='analysis-registries', version='0', type=notebook, updated_at=2024-03-28 10:22:41 UTC, created_by_id=1)
π£ run: Run(uid='SebWd1hkmKyITL1cxdEa', started_at=2024-03-28 10:22:41 UTC, is_consecutive=True, transform_id=1, created_by_id=1)
π€ created_by: User(uid='DzTjkKse', handle='testuser1', name='Test User1', updated_at=2024-03-28 10:21:44 UTC)
Features:
var: FeatureSet(uid='rl3fIg1A12AdQ06M6vTy', n=11277, type='number', registry='bionty.Gene', hash='JPtZ0oY6iau9nhXrfUJl', updated_at=2024-03-28 10:25:36 UTC, created_by_id=1)
'BCAS2', 'CCNE2', 'TMEM120B', 'RNF31', 'RNF31', 'STK17B', 'DOK4', 'B3GNTL1', 'B3GNTL1', 'CCND1', 'PSMG2', 'SLC25A42', 'DGAT2', 'CXCL1', 'UBB', 'RFXAP', 'UBE2E3', 'GMEB1', 'BTRC', 'ACSM1', ...
obs: FeatureSet(uid='zmZPhlm7fQrAdght3xO0', n=2, registry='core.Feature', hash='in8Toe8fLtWaB0-5_q7l', updated_at=2024-03-28 10:25:37 UTC, created_by_id=1)
π stim (2, core.ULabel): 'STIM', 'CTRL'
π cell_type_celltypist (1, bionty.CellType): 'plasmacytoid dendritic cell'
STIM-up-DEGs: FeatureSet(uid='A43gQCkY6NV4Wx2xoayU', name='Up-regulated DEGs STIM vs CTRL', n=660, type='category', registry='bionty.Gene', hash='wDH5mY0g4PdFcMAKTXeV', updated_at=2024-03-28 10:25:38 UTC, created_by_id=1)
'IFI35', 'HLA-B', 'HLA-B', 'HLA-B', 'HLA-B', 'HLA-B', 'HLA-B', 'GTF2B', 'ODF2L', 'C3AR1', 'RRAGC', 'CCDC85B', 'DNAJB4', 'PSMG2', 'GBP1', 'STAT3', 'PIM2', 'PIM2', 'ARHGAP25', 'LAG3', ...
STIM-down-DEGs: FeatureSet(uid='jZMcXblkx03q27WbPfmB', name='Down-regulated DEGs STIM vs CTRL', n=1095, type='category', registry='bionty.Gene', hash='ctJh262NyEi3bitctZvZ', updated_at=2024-03-28 10:25:38 UTC, created_by_id=1)
'MAD2L2', 'TMEM14B', 'SDC2', 'PPP1R7', 'CXCL1', 'UFC1', 'DAZAP1', 'PSMD9', 'ATP5F1D', 'TECR', 'PAIP2', 'MTSS1', 'HSD17B10', 'ZNHIT3', 'GET3', 'BTG1', 'ZNHIT3', 'ATP5PF', 'RPL29', 'CD44', ...
Labels:
π·οΈ cell_types (1, bionty.CellType): 'plasmacytoid dendritic cell'
π·οΈ ulabels (2, core.ULabel): 'STIM', 'CTRL'
Querying cell types#
Querying for cell types contains βB cellβ in the name:
bt.CellType.filter(name__contains="B cell").df().head()
uid | name | ontology_id | abbr | synonyms | description | created_at | updated_at | public_source_id | created_by_id | |
---|---|---|---|---|---|---|---|---|---|---|
id |
Querying for all artifacts annotated with a cell type:
celltypes = bt.CellType.lookup()
celltypes.plasmacytoid_dendritic_cell
CellType(uid='3JO0EdVd', name='plasmacytoid dendritic cell', ontology_id='CL:0000784', synonyms='type 2 DC|pDC|interferon-producing cell|IPC|T-associated plasma cell|plasmacytoid T cell|DC2|plasmacytoid monocyte|lymphoid dendritic cell', description='A Dendritic Cell Type Of Distinct Morphology, Localization, And Surface Marker Expression (Cd123-Positive) From Other Dendritic Cell Types And Associated With Early Stage Immune Responses, Particularly The Release Of Physiologically Abundant Amounts Of Type I Interferons In Response To Infection.', updated_at=2024-03-28 10:25:16 UTC, public_source_id=21, created_by_id=1)
ln.Artifact.filter(cell_types=celltypes.plasmacytoid_dendritic_cell).df()
uid | storage_id | key | suffix | accessor | description | version | size | hash | hash_type | n_objects | n_observations | transform_id | run_id | visibility | key_is_virtual | created_at | updated_at | created_by_id | |
---|---|---|---|---|---|---|---|---|---|---|---|---|---|---|---|---|---|---|---|
id | |||||||||||||||||||
1 | iNMvFYSmZNYKZBWcAWJN | 1 | None | .h5ad | AnnData | seurat_ifnb_activated_Bcells | None | 215062782 | Py_jXTAbsMikdIQYeoOyGv | sha1-fl | None | None | 1 | 1 | 1 | True | 2024-03-28 10:25:35.770916+00:00 | 2024-03-28 10:25:37.221230+00:00 | 1 |
Querying pathways#
Querying for pathways contains βinterferon-betaβ in the name:
bt.Pathway.filter(name__contains="interferon-beta").df()
uid | name | ontology_id | abbr | synonyms | description | public_source_id | created_at | updated_at | created_by_id | |
---|---|---|---|---|---|---|---|---|---|---|
id | ||||||||||
684 | 1l4z0v8W | cellular response to interferon-beta | GO:0035458 | None | cellular response to fibroblast interferon|cel... | Any Process That Results In A Change In State ... | 47 | 2024-03-28 10:22:04.375377+00:00 | 2024-03-28 10:22:04.375386+00:00 | 1 |
2130 | 1NzHDJDi | negative regulation of interferon-beta production | GO:0032688 | None | down regulation of interferon-beta production|... | Any Process That Stops, Prevents, Or Reduces T... | 47 | 2024-03-28 10:22:04.521279+00:00 | 2024-03-28 10:22:04.521288+00:00 | 1 |
3127 | 3x0xmK1y | positive regulation of interferon-beta production | GO:0032728 | None | positive regulation of IFN-beta production|up-... | Any Process That Activates Or Increases The Fr... | 47 | 2024-03-28 10:22:04.728280+00:00 | 2024-03-28 10:22:04.728289+00:00 | 1 |
4334 | 54R2a0el | regulation of interferon-beta production | GO:0032648 | None | regulation of IFN-beta production | Any Process That Modulates The Frequency, Rate... | 47 | 2024-03-28 10:22:04.852269+00:00 | 2024-03-28 10:22:04.852278+00:00 | 1 |
4953 | 3VZq4dMe | response to interferon-beta | GO:0035456 | None | response to fiblaferon|response to fibroblast ... | Any Process That Results In A Change In State ... | 47 | 2024-03-28 10:22:04.917860+00:00 | 2024-03-28 10:22:04.917870+00:00 | 1 |
Query pathways from a gene:
bt.Pathway.filter(genes__symbol="KIR2DL1").df()
uid | name | ontology_id | abbr | synonyms | description | public_source_id | created_at | updated_at | created_by_id | |
---|---|---|---|---|---|---|---|---|---|---|
id | ||||||||||
1346 | 7S7qlEkG | immune response-inhibiting cell surface recept... | GO:0002767 | None | immune response-inhibiting cell surface recept... | The Series Of Molecular Signals Initiated By A... | 47 | 2024-03-28 10:22:04.442240+00:00 | 2024-03-28 10:22:04.442249+00:00 | 1 |
Query artifacts from a pathway:
ln.Artifact.filter(feature_sets__pathways__name__icontains="interferon-beta").first()
Artifact(uid='iNMvFYSmZNYKZBWcAWJN', suffix='.h5ad', accessor='AnnData', description='seurat_ifnb_activated_Bcells', size=215062782, hash='Py_jXTAbsMikdIQYeoOyGv', hash_type='sha1-fl', visibility=1, key_is_virtual=True, updated_at=2024-03-28 10:25:37 UTC, storage_id=1, transform_id=1, run_id=1, created_by_id=1)
Query featuresets from a pathway to learn from which geneset this pathway was computed:
pathway = bt.Pathway.filter(ontology_id="GO:0035456").one()
pathway
Pathway(uid='3VZq4dMe', name='response to interferon-beta', ontology_id='GO:0035456', synonyms='response to fiblaferon|response to fibroblast interferon|response to interferon beta', description='Any Process That Results In A Change In State Or Activity Of A Cell Or An Organism (In Terms Of Movement, Secretion, Enzyme Production, Gene Expression, Etc.) As A Result Of An Interferon-Beta Stimulus. Interferon-Beta Is A Type I Interferon.', updated_at=2024-03-28 10:22:04 UTC, public_source_id=47, created_by_id=1)
degs = ln.FeatureSet.filter(pathways__ontology_id=pathway.ontology_id).one()
Now we can get the list of genes that are differentially expressed and belong to this pathway:
contributing_genes = pathway.genes.all() & degs.genes.all()
contributing_genes.list("symbol")
['CALM1',
'PNPT1',
'IFITM1',
'OAS1',
'STAT1',
'XAF1',
'SHFL',
'IFI16',
'PLSCR1',
'IFITM2',
'IFITM3',
'AIM2',
'IRF1',
'BST2',
'MNDA']
# clean up test instance
!lamin delete --force use-cases-registries
!rm -r ./use-cases-registries
Show code cell output
π‘ deleting instance testuser1/use-cases-registries
β manually delete your stored data: /home/runner/work/lamin-usecases/lamin-usecases/docs/use-cases-registries